Factor Analysis:
Exploratory research on a topic may identify many variables of possible interest, so many that their sheer number can become a hindrance to effective and efficient analysis.
Factor analysis is a "data reduction" technique that reduce the number of variables studied to a more limited number of underlying "factors."
Factor analysis is based on a model that supposes that correlations between pairs of measured variables can be explained by the connections of the measured variables to a small number of non-measurable (latent), but meaningful variables, which are termed factors.
The aims of factor analysis are to: (i) identify the number of factors; (ii) define the factors as functions of the measured variables; (iii) study the factors which have been defined.
Statistics Center
Logo Statistics Center
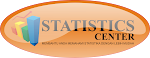
STATISTICS CENTER
Statistics Center (SCe) merupakan suatu lembaga yang bergerak di bidang jasa Analisis Data dan Pelatihan Software Statistika (SPSS, MINITAB, E-Views, SAS, dll). SCe secara resmi didirikan pada maret 2009. Berawal dari adanya Program Mahasiswa Wirausaha (PMW) yang diadakan oleh Universitas Brawijaya, Sce akhirnya lulus seleksi bisnis plan tingkat Fakultas hingga Universitas (Total bisnis plan yang masuk 200 lebih disaring menjadi 65 bisnis plan).
Berdasarkan hasil survey yang telah dilakukan pada beberapa mahasiswa, 75% responden menyatakan bahwa statistika itu sulit dan cukup mahalnya biaya untuk melakukan analisis data pada beberapa lembaga analisis di kota malang membuat enggan mahasiswa untuk menggunakan layanan ini . Untuk itu, rencana dalam program ini kami ingin mendirikan lembaga di bidang jasa konsultasi statistika yang bernama ”Statistics Center (SCe)”. Lembaga ini diharapkan mampu membantu menyelesaikan masalah-masalah yang ada khususnya dalam hal penelitian baik dari akademisi, instansi pemerintah maupun swasta.
Berdasarkan hasil survey yang telah dilakukan pada beberapa mahasiswa, 75% responden menyatakan bahwa statistika itu sulit dan cukup mahalnya biaya untuk melakukan analisis data pada beberapa lembaga analisis di kota malang membuat enggan mahasiswa untuk menggunakan layanan ini . Untuk itu, rencana dalam program ini kami ingin mendirikan lembaga di bidang jasa konsultasi statistika yang bernama ”Statistics Center (SCe)”. Lembaga ini diharapkan mampu membantu menyelesaikan masalah-masalah yang ada khususnya dalam hal penelitian baik dari akademisi, instansi pemerintah maupun swasta.
Factor Analysis
Diposting oleh
Statistics Center
on Rabu, 22 Juli 2009
Label:
analisis data,
Factor Analysis
/
Comments: (1)
Cluster Analysis
Diposting oleh
Statistics Center
Label:
analisis data,
Cluster Analysis
/
Comments: (0)
Cluster Analysis:
In multivariate analysis, cluster analysis refers to methods used to divide up objects into similar groups, or, more precisely, groups whose members are all close to one another on various dimensions being measured. In cluster analysis, one does not start with any apriori notion of group characteristics. The definition of clusters emerges entirely from the cluster analysis - i.e. from the process of identifying "clumps" of objects.
In multivariate analysis, cluster analysis refers to methods used to divide up objects into similar groups, or, more precisely, groups whose members are all close to one another on various dimensions being measured. In cluster analysis, one does not start with any apriori notion of group characteristics. The definition of clusters emerges entirely from the cluster analysis - i.e. from the process of identifying "clumps" of objects.
Hypothesis testing
Diposting oleh
Statistics Center
Label:
analisis data,
hypothesis testing,
Statistics Center
/
Comments: (0)
Hypothesis testing (also called "significance testing") is a statistical procedure for discriminating between two statistical hypotheses - the null hypothesis (H0) and the alternative hypothesis ( Ha, often denoted as H1). Hypothesis testing rests on the presumption of validity of the null hypothesis - that is, the null hypothesis is accepted unless the data at hand testify strongly enough against it.
The philosophical basis for hypothesis testing lies in the fact that random variation pervades all aspects of life, and in the desire to avoid being fooled by what might be chance variation. The alternative hypothesis typically describes some change or effect that you expect or hope to see confirmed by data. For example, new drug A works better than standard drug B. Or the accuracy of a new weapon targeting system is better than historical standards. The null hypothesis embodies the presumption that nothing has changed, or that there is no difference.
Hypothesis testing comes into play if the observed data do, in fact, suggest that the alternative hypothesis is true (the new drug produces better survival times than the old one in an experiment, for example). We ask the question "is it possible that chance variation might have produced this result?"
As noted, the null hypothesis stands ("is accepted") unless the data at hand provide strong enough evidence against it. "Strong enough" means that probability that you would obtain a result as extreme as the observed result, given that the null hypothesis is true, is small enough (usually < 0.05) given the null hypothesis is true.
The philosophical basis for hypothesis testing lies in the fact that random variation pervades all aspects of life, and in the desire to avoid being fooled by what might be chance variation. The alternative hypothesis typically describes some change or effect that you expect or hope to see confirmed by data. For example, new drug A works better than standard drug B. Or the accuracy of a new weapon targeting system is better than historical standards. The null hypothesis embodies the presumption that nothing has changed, or that there is no difference.
Hypothesis testing comes into play if the observed data do, in fact, suggest that the alternative hypothesis is true (the new drug produces better survival times than the old one in an experiment, for example). We ask the question "is it possible that chance variation might have produced this result?"
As noted, the null hypothesis stands ("is accepted") unless the data at hand provide strong enough evidence against it. "Strong enough" means that probability that you would obtain a result as extreme as the observed result, given that the null hypothesis is true, is small enough (usually < 0.05) given the null hypothesis is true.